Kenway Louie
The vision scientist David Marr noted that information processing can be understood at three fundamental levels: at the level of functional goal (computational), at the level of representation (algorithmic), and at the level of instantiation (physical). Thus, understanding a neural system requires a broader knowledge of both what problem the system aims to solve and the form of possible solutions. I am interested in the algorithmic-level processing of neural decision circuits, and how such representations mediate the translation between brain activity and behavior. In particular, my work focuses on value-related activity in the frontal and parietal circuits that control the selection of saccadic eye movements.
One of my primary interests is quantifying the neural code governing the representation of value information. Such information is critical to the decision process, guiding the choice between potential options and bridging the gap between sensation and action. We have recently shown that value coding in parietal cortex is relative rather than absolute, imparting an intrinsic context-dependence to value representation. This relative representation is governed by divisive normalization, a computational algorithm widely described in sensory cortices, suggesting a common cortical mechanism for contextual processing.
Normalized value coding provides insight into both the physiological mechanism of decision-making and the efficiency of choice behavior. Using computational modeling and human choice experiments, we find that the the combination of normalization and stochastic variability in neural value coding can explain many context-dependent violations of rational choice theory. Ongoing projects include the development of normalization-based behavioral strategies to remediate context-dependent choice inefficiencies, examining value coding in human subjects using neuroimaging techniques, and extending the normalization framework to the temporal domain by investigating adaptation in value coding.
Future research goals include the examination of decision-making as a dynamical system and a characterization of the efficiency of the neural value code. Ultimately, understanding the representational level of decision-making will require a fuller knowledge of the dynamic behavior of the large number of interacting neurons in relevant cortical circuits, which we are addressing via simultaneous recording of frontoparietal neurons during value-guided choice. Equally important is an understanding of the constraints inherent in neural information processing. Divisive normalization mediates efficient coding in sensory systems, suggesting that value representation may reflect an analogous optimization in the neural code for value.
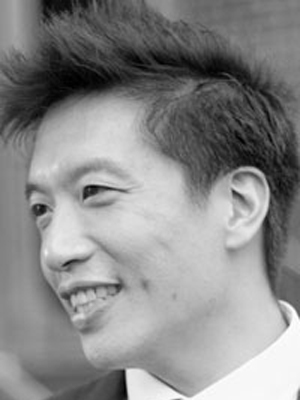